Imagine a world where doctors can see a vivid and detailed 3D representation of your heart, all generated from a single ultrasound image. That might sound like a plot from a sci-fi movie, but thanks to recent breakthroughs in medical technology, it’s rapidly becoming a reality. At the heart of this revolution is the International Conference on Medical Image Computing and Computer Assisted Intervention (MICCAI). This prestigious gathering serves as a global stage where cutting-edge advances in imaging sciences and healthcare come to light. In its 2023 iteration, MICCAI introduced a special feature – ASMUS 23, a dedicated symposium highlighting the significant strides AI and Machine Learning (ML) are making in the realm of medical ultrasound. [ Proceedings ].
Diving right in, let’s explore the first two interesting papers from this symposium:
Echo from Noise: Synthetic Ultrasound Image Generation Using Diffusion Models for Real Image Segmentation
Authors: David Stojanovski, Uxio Hermida , Pablo Lamata, Arian Beqiri and Alberto Gomez
In the complex world of medical imaging, the challenge of annotating images is one that many businesses face. Think about the painstaking process of detailing every curve and contour of multiple objects within a single image. Now, contrast this with merely tagging the image under a category. To bring this to life, consider the image below. It provides a snapshot of a four-chamber view from an ultrasound, captured at different moments in time.
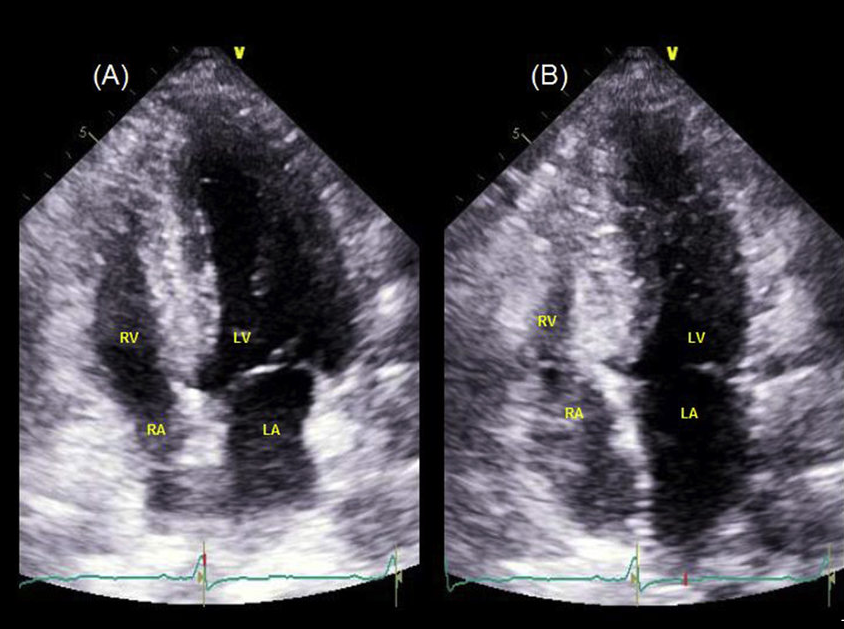
End of Diastole
Left ventricle (LV) and right ventricle (RV) are at the maximum volume because they are filled with blood. The mitral and tricuspid valves are open, allowing blood to fill the ventricles from the atria. Both atria (LA, RA) are relatively empty as they’ve just pushed blood into the ventricles.
End of Systole (just after the heart contracts):
Both ventricles (LV and RV) have ejected a significant portion of their blood. The LV pumps blood into the aorta, distributing it to the body, while the RV pumps blood into the pulmonary artery, directing it to the lungs for oxygenation. At this point, the mitral and tricuspid valves are closed to prevent the backflow of blood to the atria. Meanwhile, the aortic and pulmonic valves are open to allow the ejection of blood. The atria are starting to fill with blood again. The LA receives oxygenated blood returning from the lungs via the pulmonary veins, and the RA gets deoxygenated blood from the body via the superior and inferior vena cava.
So, what did the authors propose?
Semantic diffusion model that can create the whole ultrasound based on available segmentation labels. Now, we can use simple data augmentation / elastic deformation to create new labels (without corresponding images). Then, the SDM generates the synthetic ultrasound that can later be used for training neural networks. The authors claim a 13% improvement over the state of the results.
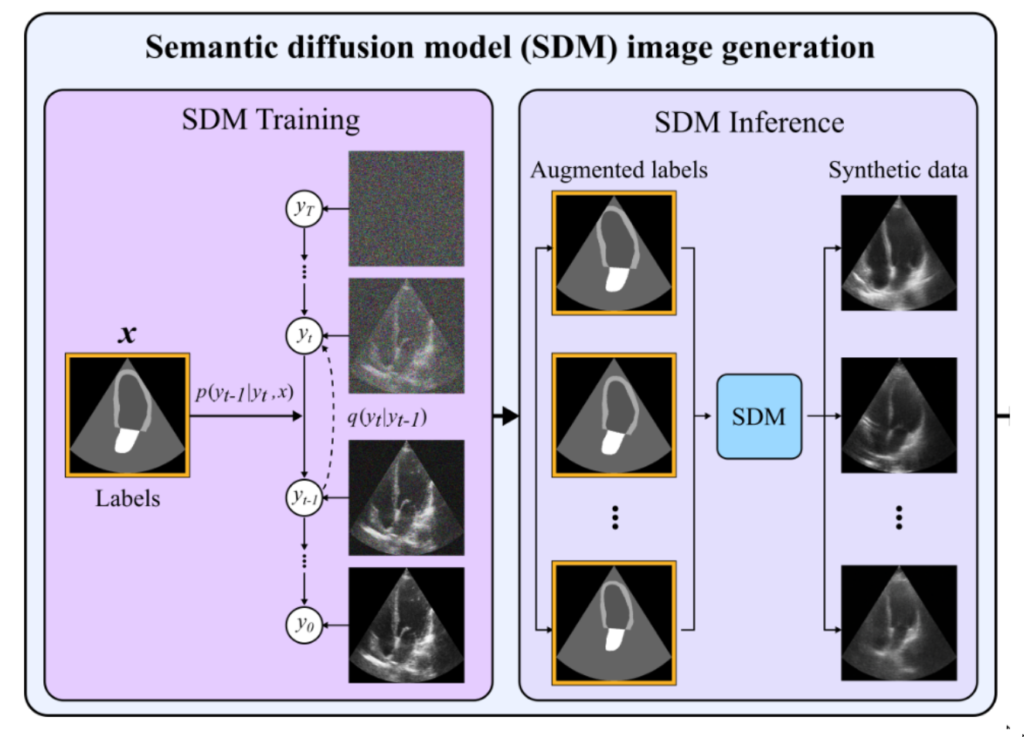
Now, imagine, would you like to, as a certified physician, annotate every slice of the heart cycle for who knows how many cycles and how many patients? What if there was a way to automate this intricate process, saving precious time while ensuring accuracy?
Graph Convolutional Neural Networks for Automated Echocardiography View Recognition: A Holistic Approach
Authors: Sarina Thomas, Cristiana Tiago, Børge Solli Andreassen, Svein-Arne Aase, Jurica Šprem, Erik Steen, Anne Solberg & Guy Ben-Yosef
Cardiac ultrasound (US) plays an instrumental role in the diagnosis and management of various heart conditions. The heart, with its intricate anatomy and dynamic function, is conventionally assessed using several standard views, which serve as pivotal reference points for precise diagnostic evaluations. Automatic view recognition, while simplifying this process, faces challenges in ensuring that the images are perfectly poised for diagnostic measurements. This involves not just classifying these views but also ensuring the image’s accuracy in terms of the heart’s location, orientation, and the absence of obstructions. Recent strides in deep learning have made significant improvements, but there still exists a gap in assuring the ideal suitability of an image for specific diagnostic purposes.
Imagine you’re a physician and you want to detect views. For Transesophageal Echocardiography (TEE), there are more than 24 standardized views! Just remembering how they look must be difficult, not to navigate ultrasound probe within the body. In other words, the researchers want to make it easier and more precise to diagnose heart conditions using ultrasound images. Instead of just telling what part of the heart we’re looking at, they’re trying to make sure that:
- The right structures of the heart are in the image.
- We can see these structures clearly without any obstructions.
- The image is taken from the best angle for accurate diagnosis.
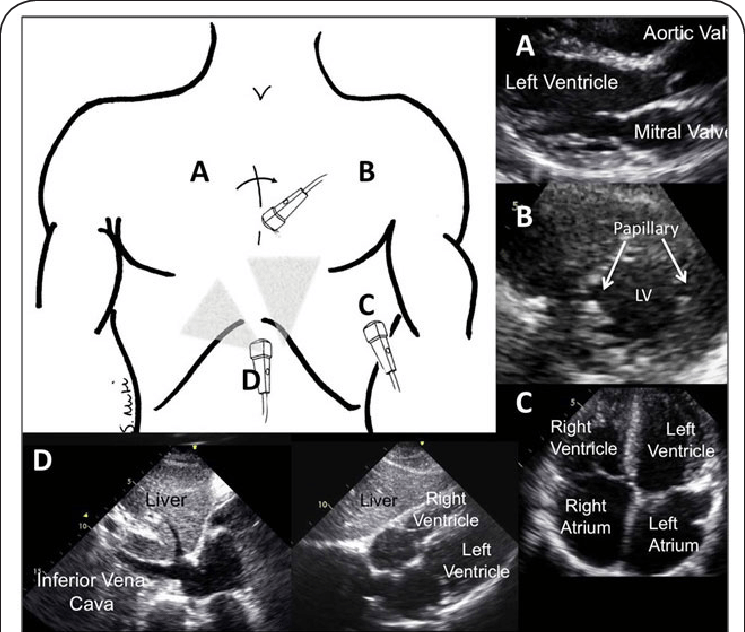
Imagine the heart as a 3D model inside the body. Now, when doctors take an ultrasound image, they’re essentially taking a 2D ‘slice’ of this 3D model. The researchers are trying to predict where this slice is taken from in the 3D model. The team uses a digital 3D model of the heart, similar to a mesh made up of points (vertices) connected with lines. These models are taken from actual patient data and adjusted to have the same number of points for consistency. To teach their system how different ‘slices’ of the heart look, they generated 2D images based on their 3D model. These images replicate the way a cardiologist would view the heart using an ultrasound. They then added features to make the images look more like actual ultrasound scans.
Because they didn’t have a complete dataset with all the details they needed, the team turned to a technique called a diffusion model. This model can create very realistic ultrasound images from the data they generated earlier. It’s a bit like an artist starting with a rough sketch (from their generated data) and then adding layers of details to make it look like a real picture.
At the heart (pun intended) of their method is something called a Graph Neural Network (GCN). If you picture the 3D heart model as a connected web or network, this GCN can process information from this network. Unlike regular image processing, which is often done on flat, 2D images, a GCN can handle the complex structure of the 3D heart model. It takes the realistic ultrasound images, processes them, and tries to determine the exact position and orientation of the 2D ‘slice’ in the 3D model.
Share your thoughts on how AI could revolutionize other areas of healthcare or if there are potential drawbacks we should be wary of.
Brilliant!!